Machine Learning, artificial intelligence serving the renewable energies
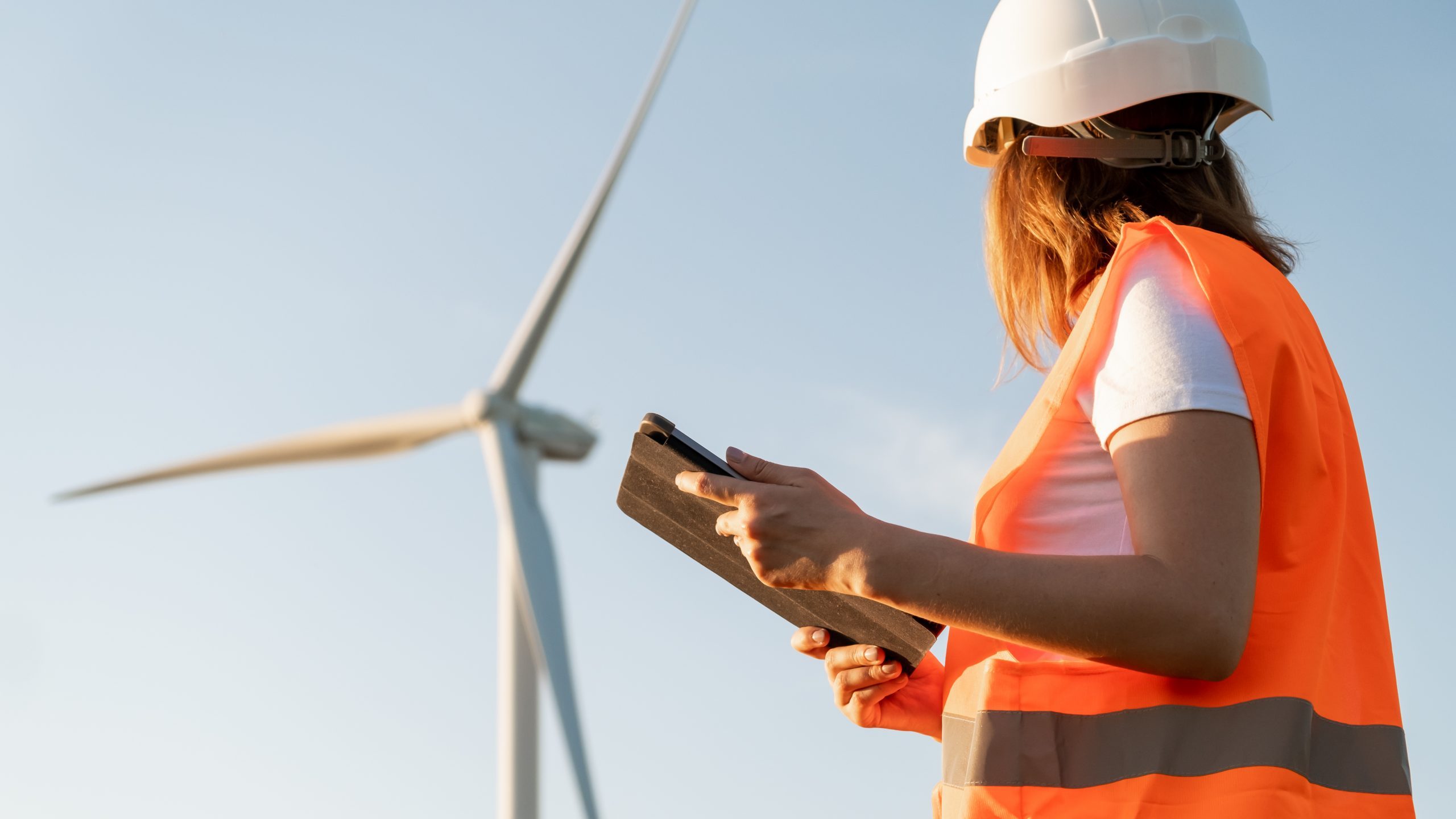
Machine Learning, artificial intelligence serving the renewable energies
By Fernando Heredia – Source: Energía Online
This is a tool used in the entire world that is starting to settle in Argentina. It can predict faults in equipment and increase park generation more than 2%.
We are at the operating system of Genneia. Patricio Neffa, the Director of Innovation, Development and Project Management, picks up one of the 236 turbines that are functioning throughout the different wind parks across the country and starts checking its vital signs: the system of the hydraulic group, the Pitch regulation, the multiplier, the generator and the transformer, as he were a doctor examining his patient.
While he examines the data, he explains, “From here, Olivos, I am able to monitor, operate and supervise everything happening in all our plants in the country, and if I would like to, I can turn switches on and off with just a click and command the operation of our plant which is about 1200 km far away”.
However, this advanced and independent SCADA tool, which is used by very few Argentine companies, still requires a complex processing of all this information, which impedes the necessary automation to make key decisions that anticipate the frequent faults of machines. This problem has been gone in depth in Argentina because of its remote location and its obstacles to import some pieces.
[Genneia and PAE opened the Chubut Norte III Wind Park]
Sometimes, the replacement of a spare part can take about four months between the time the import is authorized, the ship arrives from Europe or China, Customs approves the permit, and it is shipped to Patagonia. A whole period in which that panel or mill could not generate energy and caused a significant loss of income.
"The person who may put itself in my shoes must control 617 MW of operational wind power, which is equivalent to 236 turbines, plus 280 thousand solar panels, 6 gas turbines, 11 inverters, switching stations and many other things. It is like having a huge fleet of cars of different brands and models to do the work. It is not the same. And on top of that, you have them 1200 km away. All this adds up to a very important complexity to solve", Patricio graphs.
“Machine Learning may increase the generation of a park by 2%, which could represent an additional $350,000 to $500,000 per year”.
That’s why in Genneia, a pilot test has been started this year to incorporate Machine Learning, the technology that is shaking the renewable parks management up in the most developed countries.
"Machine Learning means teaching a machine or algorithm to perform tasks. The difference between the traditional paradigm and Machine Learning is that we will never tell the computer exactly what to do. We will let it discover patterns or give it samples of what we want'', Tomás Criado, CEO of Epical and Board Member of Atome, one of the Argentine providers of Machine Learning tools, tells EOL.
To give an idea of the impact that Machine Learning can have on the industry, Atome estimates that, thanks to wind forecasts, failure prevention and maintenance optimization, Machine Learning can increase the generation of a wind farm by 2% "with a conservative criterion" which, for a 100 MW farm and a load factor of 50%, would represent an additional income of between $350,000 and $500,000 per year.
[The generation through renewable energy reached an interannual growth of 52%]
In Genneia, the added value of this technology, developed by the company Isotrol, is measured as "avoided loss of production", i.e., the possibility of avoiding production losses due to idle equipment. If during this period of inactivity the sufficient wind or solar resources are assumed, they may save between 2.5% and 3.5% of annual energy production in losses. "We have found that in a year and a half we recover the investment made in incorporating this tool. Over the 20-year useful life of a wind farm, translated into monetary value, the benefits are millions of dollars," says Neffa.

He adds, "This is the first time this has been done in the country. We are one of the few that have an independent SCADA to manage renewable assets and practically the only ones that use the digital asset management tools with Machine Learning of this SCADA. The Madryn pilot was very successful (0.5% energy recovery or the equivalent of 9.6% avoided loss of production) and now we need to take it to all the company's assets”.
How does Machine Learning work?
Advanced data analysis through the application of Machine Learning techniques makes it possible to treat a huge volume of data, process it in real time and generate information to make a decision that anticipates a failure. To do this, the algorithms compare the operating performance of a solar panel or a wind turbine with the normal operating pattern, detecting deviations and indicating the probable causes to the operator.
[Smart grids, the future nets are already all the rage in the world]
Neffa says, "The tool will learn from behavior patterns and will point out deviations without you having to go in and check each of the stages. It tells you two possible causes and generates a recommendation with an 80% probability”. In addition, he lists some of the most frequent problems that can be detected before they have an impact on electricity production, which are a blocked tracker, overheating of a generator or dirt on the panels.
The importance of weather forecasting
Before building a wind farm, generators install wind measurement towers of 80/120 meters to carry out studies for one to two years and check the potential of the resource in the medium and long term. But once the farm is operating, they work with four- to five-day weather forecasts that will provide key information to combine with Machine Learning tools.
"We have a degree of reliability of 95% for the next three days and 90% for the next five. And if, combined with Machine Learning, you already know how the turbines are performing, it becomes a huge generation forecasting tool. It is vital data that allows us to know how much energy we are going to generate and gives us a great financial forecast", Genneia assures.
At the same time, knowing the precise moment when the wind will not blow, gives you the possibility to plan, at the right time, the repair of those machines in which Machine Learning detected failures. This way, no production hours are wasted. As the wind is fluctuating and on the same day you can have a peak and a trough, the most efficient way is to take advantage of the valleys to take a machine out of operation.